Release time:2022-06-01 Hits:
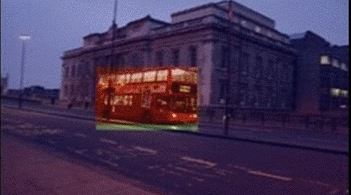
- Indexed by:Journal paper
- Document Code:s11063-022-10855-0
- First Author:Xiao Wang
- Correspondence Author:Baochang Zhang
- Co-author:Xiang Xiang,Xuhui Liu,Jianying Zheng,QingLei Hu
- Journal:Neural Processing Letters
- Included Journals:SCI、EI
- Affiliation of Author(s):Springer Nature
- Place of Publication:Belgium
- Discipline:Engineering
- Document Type:J
- Key Words:Neural Processing Letters
- DOI number:10.1007/s11063-022-10855-0
- Date of Publication:2022-05-30
- Impact Factor:3.0
- Abstract:Weakly supervised object detection which reduces the need for strong supervision during training has recently made significant achievements. However, it remains a challenging issue due to the time-consuming and labor-intensive problems in application. To further reduce the label cost, we introduce a new fusion method of weakly supervised learning and active learning in a unified framework for object detection. Weakly supervised learning based on min-entropy latent model is used to weaken the labels by image-label, while active learning is used to reduce the quantity of labelled images. The fusion method proposed can effectively reduce the dependency of object detection on manual annotation. In this paper, we introduce three strategies of active learning, including least confidence sampling, margining sampling and weighted classification sampling. To validate the effectiveness of each strategy and different sample compositions in weakly supervised learning object detection, we conducted lots of experiments. Extensive experiments show that the combination of image-level labeling and active learning can achieve comparable results with the previous state-of-the-art methods with much lower label cost.
- Links to published journals:https://link.springer.com/article/10.1007/s11063-022-10855-0