Release time:2022-07-06 Hits:
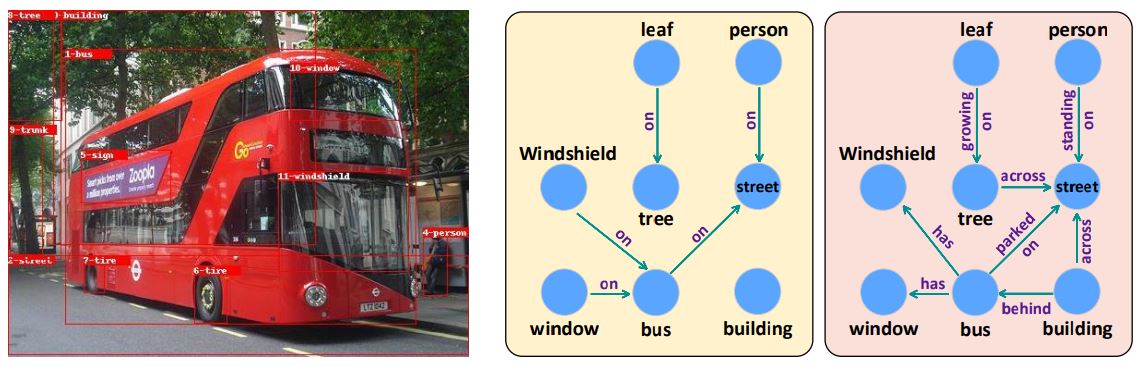
- Indexed by:Essay collection
- First Author:Youming Deng
- Co-author:Yansheng Li,Yongjun Zhang,Xiang Xiang,Jian Wang,Jingdong Chen,Jiayi Ma
- Journal:Proceedings of European Conference on Computer Vision (ECCV) 2022
- Included Journals:EI
- Place of Publication:Germany
- Discipline:Engineering
- First-Level Discipline:Control Science and Engineering
- Document Type:C
- Date of Publication:2022-07-06
- Abstract:As far as Scene Graph Generation (SGG), coarse and fine predicates mix in the dataset due to the crowd-sourced labeling, and the long-tail problem is also pronounced. Given this tricky situation, many existing SGG methods treat the predicates equally and learn the model under the supervision of mixed-granularity predicates in one stage, leading to relatively coarse predictions. In order to alleviate the negative impact of the suboptimum mixed-granularity annotation and long-tail effect problems, this paper proposes a novel Hierarchical Memory Learning (HML) framework to learn the model from simple to complex, which is similar to the human beings' hierarchical memory learning process. After the autonomous partition of coarse and fine predicates, the model is first trained on the coarse predicates and then learns the fine predicates. In order to realize this hierarchical learning pattern, this paper, for the first time, formulates the HML framework using the new Concept Reconstruction (CR) and Model Reconstruction (MR) constraints. It is worth noticing that the HML framework can be taken as one general optimization strategy to improve various SGG models, and significant improvement can be achieved on the SGG benchmark (i.e., Visual Genome).